Data Quality: The Key to Reliable AI
Ensuring data quality is crucial for successful data-driven initiatives, especially AI systems, as it underpins reliable analytics and accurate decision-making. Poor data quality leads to inaccurate predictions and biases. Key reasons for maintaining data quality in AI include enhancing accuracy, mitigating biases, and improving efficiency. Best practices involve robust data governance, regular verification and cleansing, careful feature selection, continuous monitoring, and using validated external data.
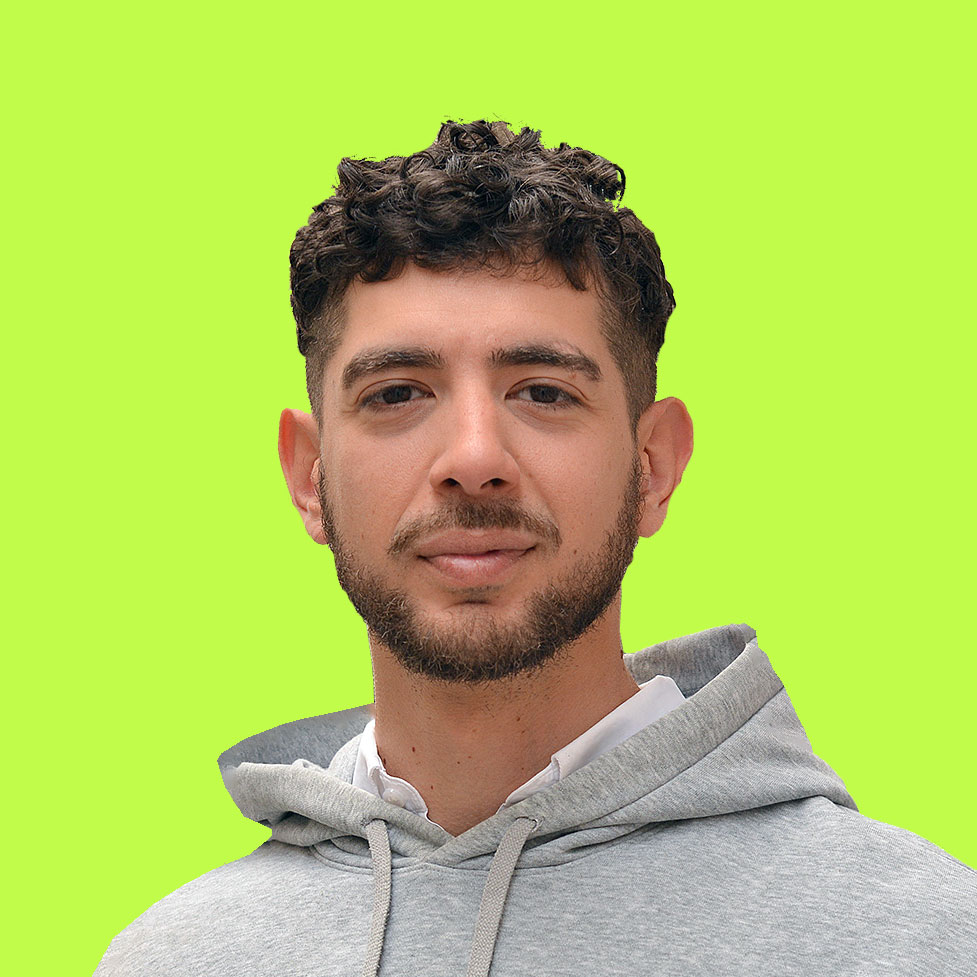
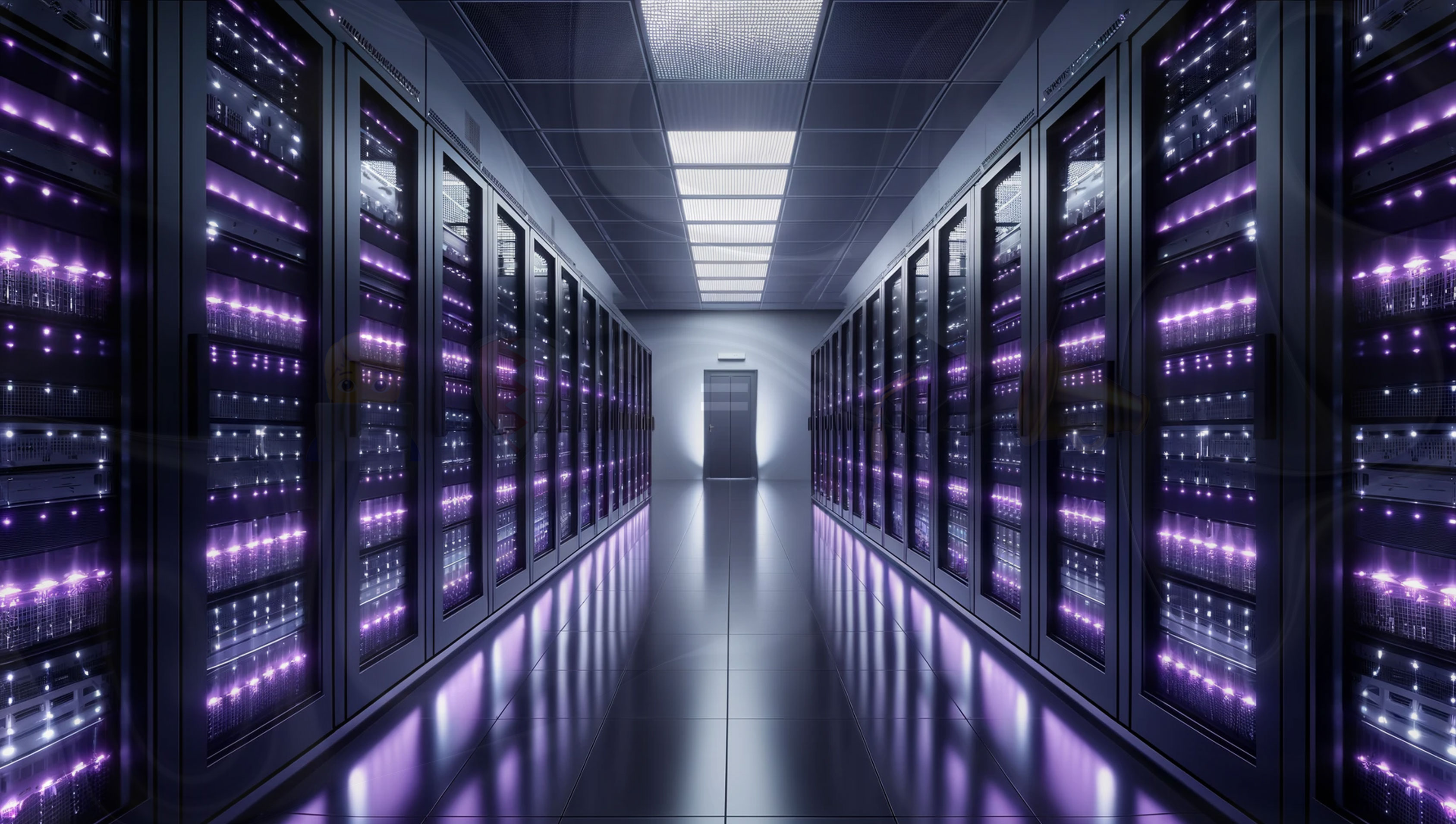
Introduction
In today’s data-driven world, ensuring data quality is crucial for the success of any initiative, especially when it comes to Artificial Intelligence (AI). High-quality data forms the foundation for reliable analytics and informed decision-making, while poor data quality can lead to erroneous conclusions and misguided strategies. Accurate and consistent data is essential for making sound business decisions, optimizing operations, and gaining competitive advantages. This blog post explores the importance of data quality, particularly for AI systems, and provides best practices to maintain it.
The Crucial Role of Data Quality in AI: Why It MattersIn the fast-paced world of technology, AI has revolutionized various industries, from healthcare to finance. However, the success of AI systems hinges on the quality of the data they are trained on. High-quality data ensures accuracy, reliability, and efficiency, while poor data quality can introduce biases and inefficiencies. The integrity of AI models is fundamentally dependent on the data they ingest, making the quality of this data a critical factor in the performance and trustworthiness of AI applications.
Why Data Quality Matters for AI
- Accuracy and Reliability: High-quality data enables AI models to make accurate predictions and informed decisions. Ensuring data is clean, accurate, and reliable allows AI systems to deliver valuable insights and perform tasks effectively, which is crucial for applications ranging from healthcare diagnostics to financial forecasting.
- Bias Mitigation: Ensuring balanced and representative training data helps prevent biases in AI models. Poor data quality can introduce and perpetuate biases, leading to unfair and inaccurate outcomes. Properly curated and diverse datasets are essential to promote fairness and equity in AI systems, minimizing the risk of discriminatory practices and enhancing the overall reliability of AI-driven decisions.
The Consequences of Poor Data Quality
The adage "garbage in, garbage out" highlights the impact of poor data quality on AI systems. Consequences include:
- Inaccurate Predictions: Leading to potentially serious implications in critical areas like healthcare and finance.
- Bias and Discrimination: Biased data can perpetuate inequalities and lead to unfair outcomes.
- Resource Wastage: Correcting data quality issues post-deployment can be costly and time-consuming.
Best Practices for Ensuring High-Quality Data
To harness AI's full potential, organizations must prioritize data quality. Here are some best practices:
- Data Governance: Establish a robust framework outlining standards and processes for data management to maintain consistency.
- Data Verification and Cleansing: Regularly identify and correct errors and inconsistencies to keep data accurate and up-to-date.
- Feature Selection: Carefully select relevant data points to avoid introducing biases or confusing the AI model.
- Continuous Monitoring and Improvement: Regularly monitor data quality and update AI models with accurate data reflecting real-world scenarios.
- External Data Sources: Use validated third-party datasets to complement internal data and provide additional insights.
Conclusion
In the AI revolution, data quality is the cornerstone of successful AI systems. High-quality data ensures AI models are accurate, reliable, and fair, enabling them to deliver meaningful insights and support informed decision-making. By prioritizing data quality and implementing best practices, organizations can unlock AI's full potential and drive innovation across industries. In the world of AI, quality data is not just a preference—it's a necessity. Investing in data quality today paves the way for a smarter, more efficient, and fairer tomorrow.
Other Posts
Assess your AI Maturity. Increase your organizations AI Value
Understand your organization's current maturity level & find out how to create more AI Value.